- Blog
Causal Inference for Decision-Making
Causal Inference
Understanding cause and effect is critical for strategic and organizational decision-making. Current machine learning approaches remain purely based on correlation and prediction, and are limited to analytical insights that only partially address various management decision-making problems. To generate and evaluate alternative strategic actions in terms of their effect on central business metrics, managers need to understand the causal mechanisms underlying a situation. In other words, assessing the likely impact of these interventions ex-ante requires the use of causal inference.
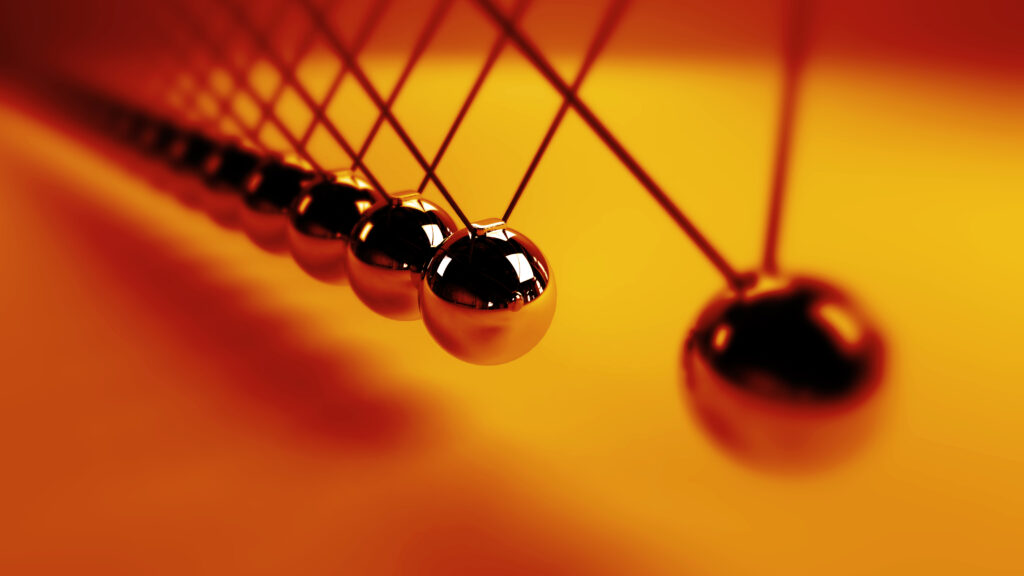
What is Causal Inference?
Every year, before Black Friday, retailers fight for customers’ attention. This results in an increased marketing budget – which is lavishly spent on TV ads. As it’s natural to expect, sales also increase dramatically – so there must be a large ROI in the ads, right?
This is in fact a complicated problem to tackle, for the following reason: around thanksgiving customers are already likely to be buying more, regardless. So in the absence of extra advertising, we should also observe an increase in sales numbers. So the question is: what is the incremental effect of the advertising spend?
In order to correctly answer this question, one would need to duplicate reality, and in this alternative – counterfactual – universe, carry out normally without increasing the marketing budget, and measure the difference in sales.
Determining the effect of advertising is an example of a causal inference problem: the process of determining the cause and effect relationship between two variables. The good news is that causal inference – usually – doesn’t require universe-cloning machines, since there’s a whole growing field of mathematics: Causal AI, dedicated to making this process simple.
Do I need Causal Inference?
Making decisions is complicated for humans, and nearly impossible for machines. The reason why this is such a tricky problem is because making a decision always involves intervening in the system, and since every action leads to a reaction, it’s oftentimes impossible or intractable to really understand the impact of our decisions.
Take a customer retention problem: it’s not sufficient to understand who is likely to churn, we also need to know, from the levers that we can pull, which one will have the largest causal effect on retention, at the lowest cost. It’s also important to understand not only whether an intervention will have a causal impact, but also how large the effect is, since ultimately the optimal decision is that which maximizes the ROI of the interventions. Offering 100% discounts for all customers is a good way to ensure retention, but doesn’t really have a good ROI.
Ultimately, making the right decision requires evaluating a series of what-if questions: and in each one of these alternative universes, understand the causal impact of our interventions and evaluate the costs and rewards to understand what is the optimal decision: the one that will have the lowest cost and the highest rewards.
What can go wrong?
Machines usually look at past data to estimate the impact of certain interventions. That creates an interesting problem: decisions aren’t usually made in isolation, there’s always a reason why we made a decision in the past – creating statistical biases that need to be corrected in order to truly understand the impact of interventions.
- The holiday season acts as a driver for us to increase our budget: there’s a confounding effect, in which we can’t easily disentangle what’s due to the holiday season, and what’s due to our intervention. In reality, most of our decisions can be potentially confounded by external events: the economy, supply chain, geopolitical issues, the weather, etc. Therefore it’s important to take these into consideration when estimating causal effects.
- Customers who complain about the service or the product will also be receiving the highest discounts: our propensity to give a discount increases with customer dissatisfaction. Because we give discounts to customers who are already unhappy, this can lead to a case of Simpson’s paradox, in which customers who receive discounts are more likely to leave, despite the fact that discounts actually have a positive causal effect on retention.
- If we only have access to the data that we collected, we may be subject to selection bias. Take the following example: In a poll of the presidential election, the Literary Digest confidently – and wrongly – predicted that Alf Landon would beat Franklin Delano Roosevelt. Despite having a large sample size, only car and telephone owners were targeted, creating a biased sample of the population that overemphasized wealthier people. Businesses are constantly facing a similar challenge: attempting to extrapolate to the overall population only based on their existing customer base.
- People are fundamentally different, and can react in varied ways to interventions: this problem is known as heterogeneous treatment effects. This problem is particularly challenging when it comes to drug discovery and approval: while drugs may be shown to work on average when comparing control and test groups, it could be that there are different – or potentially harmful – effects for certain cohorts of the population. One notorious example is Zolpidem (Ambien), for which the ideal dosage for women was cut in half in 2013 by the FDA due to its outsized effect when compared with men. Businesses also face this challenge on a day-to-day basis: how to target campaigns to extract the maximum influence on certain groups without alienating the rest?
From A/B tests to Causal AI
As we previously alluded to, the ideal method for causal inference is to duplicate the universe and treat our twin reality as our test group, in which we performa given intervention and measure its impact over time, comparing with our own reality.
In a sense, this is what randomized control trials and A/B tests attempt to do. By randomly assigning the interventions, there’s the underlying assumption that the A and B groups are fundamentally equivalent, and thus any difference we observe between the two groups will actually be due to the intervention alone.
In reality, it’s not always feasible to perform randomized control trials, thus we need to rely on observational data, thus being subject to all the possible issues that we highlighted. The good news, however, is that it is indeed possible to overcome these issues. Causal inference can be done with methods that fall into the realm of causal AI, namely:
- Causal discovery: a series of methods that allows us to build causal graphs from observational data: representing the entirety of the causal structure of the data in a simple graph – either in an automated fashion or with the aid of domain expertise.
- Structural causal modeling & causal effect estimation: These are techniques that quantify exactly what is the impact of performing an intervention on a given variable, and how this impact percolates through the entire graph. This also makes use of classical causal inference techniques, such as front door and backdoor adjustments, Instrumental variables, propensity score matching, etc.
- Decision intelligence engines: These are techniques that allow us to extract decisions from the knowledge of causal relationships. For instance, action optimization (algorithmic recourse) outputs the optimal intervention to achieve a certain goal: maximizing ROI from a marketing campaign, improving customer retention, etc. Decision intelligence engines can also be used to monitor the outcome of our decisions: the fairness engine ensures that our decisions do not lead to any potential bias or discrimination, and the scenario planning engine helps stakeholders ensure that KPIs are robust even in stressed scenarios.
Use-cases for causal inference
Causal inference is a fundamental aspect of all decision making: finding the best possible action requires understanding what are the consequences of our interventions. It’s not always obvious, however, how that fits in every industry: while we intuitively think in terms of cause and effect, action and consequence – the message tends to get lost in translation when passed on to data teams.
What we want is to retain clients, what we get is a prediction of who is going to churn. Bridging this causality gap requires phrasing questions in the right way, and evaluating outputs based on the correct measures: it’s not about accurately predicting who is going to churn, but how many clients we managed to retain based on our recommended interventions.
Customer retention is a classical example of how thinking in terms of causal inference can immediately lead to increased value, but it’s not the only one. Some additional examples include:
- Optimal pricing and promotions: setting the price of an item requires posing a fundamental counterfactual question: if I were to increase the price of this product, would customers still buy it? How many customers would I lose in this scenario?To answer this question, it’s not only enough to look at our past data of prices vs. sales, we also need to understand how that fits within the entire environment of our industry: what are our competitors doing? How is the economy doing? Are we close to a holiday season? Only by addressing all these possible confounding relationships, we can actually get a clearer picture of the causal impact of our interventions in the customers’ behavior.
- Marketing Mix Optimization: as we discussed previously, marketing is another prime example of how causal inference is required to understand the impact of our actions. In a marketing optimization problem, we are posing the following causal question: if I were to increase the marketing budget in a given channel, what would be the additional revenue?
Similarly to pricing and promotions, we need to worry about potential confounders and biases in the historical data: our marketing budget changes through time, increasing in good economic environments – which are naturally associated with higher customer spending. We are typically advertising more during the holiday season, which is also naturally a time of higher sales. - Optimizing Manufacturing Processes: another interesting use-case of causal inference is understanding the root cause of failures in processes. Suppose we want to understand, for instance, what drives failures in a manufacturing line. There are many factors which will be associated with that: were screws too loose? Did someone misplace a component? Was the temperature wrong?
Truly finding the root causes of problems is a causal inference question: we want to answer a counterfactual question: what would have happened to the quality of my product had I decreased the temperature on the manufacturing floor? What would be the quality if I choose supplier X instead of Y?
This list is in no way extensive, and in fact causal inference is already being used at scale to improve efficiency, increase revenues and reduce costs in a variety of industries: from high frequency trading to healthcare.