How Financial Institutions Can Reduce AI Regulation Risk
8 June 2022, 20:00 GMTAt a Glance
Regulations, proposals and guidance on the use of artificial intelligence (AI) for financial institutions are arriving thick and fast.It’s increasingly clear that the current stock of machine learning algorithms are in tension with the changing regulatory landscape. In contrast, Causal AI, a new category of human-centered machine intelligence, can ease adoption of responsible AI and greatly reduce regulation riskIn this article, we take a deep dive on The Monetary Authority of Singapore’s (MAS) comprehensive FEAT principles, which are at the vanguard of this incoming wave of guidance and regulation. We outline why Causal AI is the technology that banks and insurers need to comply with FEAT.
What is FEAT?
The FEAT principles offer guidance for financial institutions on how to responsibly adopt and use AI systems. The principles were published in November 2018 with additional guidance issued in February of this year.
The principles focus on four interrelated pillars — Fairness, Ethics, Accountability, and Transparency — which combine to provide a comprehensive AI governance framework.
Like the EU’s wide-ranging AI regulations, FEAT adopts a “materiality” or “risk-tiered” approach. Meaning that the application of the principles should be calibrated to how risky a given AI decision system is. Many core financial services AI use cases are highly material — including predictive underwriting, fraud detection, credit decisioning, and customer-facing marketing.
Another theme of FEAT is that best practice AI governance should be in the DNA of the model development lifecycle, from data collection through to in-flight model diagnostics, and shouldn’t be viewed as an afterthought.
Why FEAT?
There is an AI trust crisis in the financial sector. To give one data point — 1 in 2 banks believe that not enough is understood about the unintended consequences of AI.
MAS traces this trust crisis back to the shortcomings of current machine learning, which works by extracting correlations from data. They state that concerns about machine learning include that it:
- “May not always be explainable.”
- “Could be affected by a drop off in predictive accuracy in the face of rapid changes, such as those seen during the COVID-19 pandemic.”
- “Runs the risk of accentuating biases based on the data used to train the models”.
- “Learns by memorisation and draws false patterns from limited past data, a concept known as ‘overfitting’.”
These concerns “hold back adoption at scale”. We couldn’t agree more. Better technology is needed. Across each of the FEAT pillars, we show why Causal AI is that technology.
Fairness (FEAT)
Current AI systems are known to amplify the biases of tech developers and deepen existing societal disadvantages, according to Pew Research. The problem stems from the foundations of correlation-based machine learning — in particular the assumption that the future will be very similar to the past. This assumption can lead algorithms to project historical injustices into the future.
The FEAT proposals recommend embedding fairness controls in AI systems, but they are noncommittal on which fairness criteria to implement. Another Singaporean regulator, the PDPC, has issued its own AI Governance Framework that is complementary to FEAT. The PDPC Framework recommends, “performing counterfactual fairness testing… ensuring that a model’s decisions are the same in both the real world and in a counterfactual world where attributes deemed sensitive (such as race or gender) are altered.”
Counterfactual fairness testing demands Causal AI. It is one of the most promising approaches to AI fairness — see The Alan Turing Institute’s work on counterfactual fairness, DeepMind’s research on causal Bayesian networks, and causaLens’ own research setting out a regulatory framework for developing fair AI.
FEAT recognizes that “for both banking and insurance, cross functional discussions among management, data scientists, and experts in the fields of law, ethics and compliance are critical to determining the optimal strategy for fairness in their AIDA [artificial intelligence and data analytics] systems.” Causal AI enables experts, who best understand what fairness amounts to in their business and market, to interact with and constrain models. They can do so before models have been exposed to data, ensuring the AI doesn’t learn to unfairly discriminate in deployment.
Continue learning about how Causal AI promotes fairness here.
The causaLens Decision-Making Platform leverages the most powerful counterfactual fairness tools for credit decisioning and all other ethically sensitive financial use cases.
Ethics & Accountability (FEAT)
The Ethics and Accountability principles are focussed on aligning AI systems with the firm’s ethical values, and ensuring that businesses are held accountable for their AI systems’ decisions — both internally and externally.
Let’s linger on this point of external accountability. FEAT emphasizes that data subjects should be given channels to appeal and request reviews of AI-driven decisions that affect them. Banks need to answer the question — “are recourse mechanisms available to data subjects…?”
Causal AI uniquely facilitates algorithmic recourse — this is a powerful tool that enables data subjects to identify the most resource-efficient combinations of actions they need to take to overturn an AI-driven decision. For example, an applicant whose loan is rejected can identify the most efficient actions to overturn the decision.
The causaLens Platform implements algorithmic recourse, enabling users to provide data subjects with practical and reasonable recourse at the click of a button. Learn more in this blog.
The causaLens Decision-Making Platform allows all users in the enterprise to compute optimal recourse for data subjects at the click of a button.
Transparency (FEAT)
Last but by no means least, financial institutions need transparent AI systems.
84% of financial institutions feel they are impeded by a lack of transparency or explainability in their AI systems according to The World Economic Forum. Transparency is needed “to win the trust of internal stakeholders as a precondition for AI use in production”, MAS states, as well as to de-risk AI-driven decisions. Again, we agree — in our experience, explainable AI is a 10X booster of AI adoption.
Causal AI has significant advantages over correlation-based machine learning in terms of transparency and explainability.
For one, Causal AI explanations are more intuitive. As the FEAT regulations state, “humans prefer explanations to appeal to causal structure rather than correlations”. They add that “data subjects ideally require a mix of reasoning (general explanations for their decision) and action (an understanding of how they can change a model’s behavior). Adding counterfactuals that demonstrate how a decision could be improved or changed can help data subjects grasp model behavior (e.g. “had I done X, would my outcome Y have changed?”)”. In a nutshell — a good explanation is a causal explanation.
Second, Causal AI explanations are more trustworthy. At a technical level, Causal AI models are “intrinsically interpretable”, meaning that explanations are guaranteed to be faithful (as the FEAT regulations note), whereas machine learning explanations typically involve a black box model and a second, potentially unreliable explanatory model.
Third, Causal AI explanations convey actionable insights, not just raw information. Users can probe and stress-test models by examining “what-if” scenarios, and data subjects can leverage algorithmic recourse (as we described above). This kind of tooling requires causality — put simply, you need causal knowledge to anticipate the effects of actions.
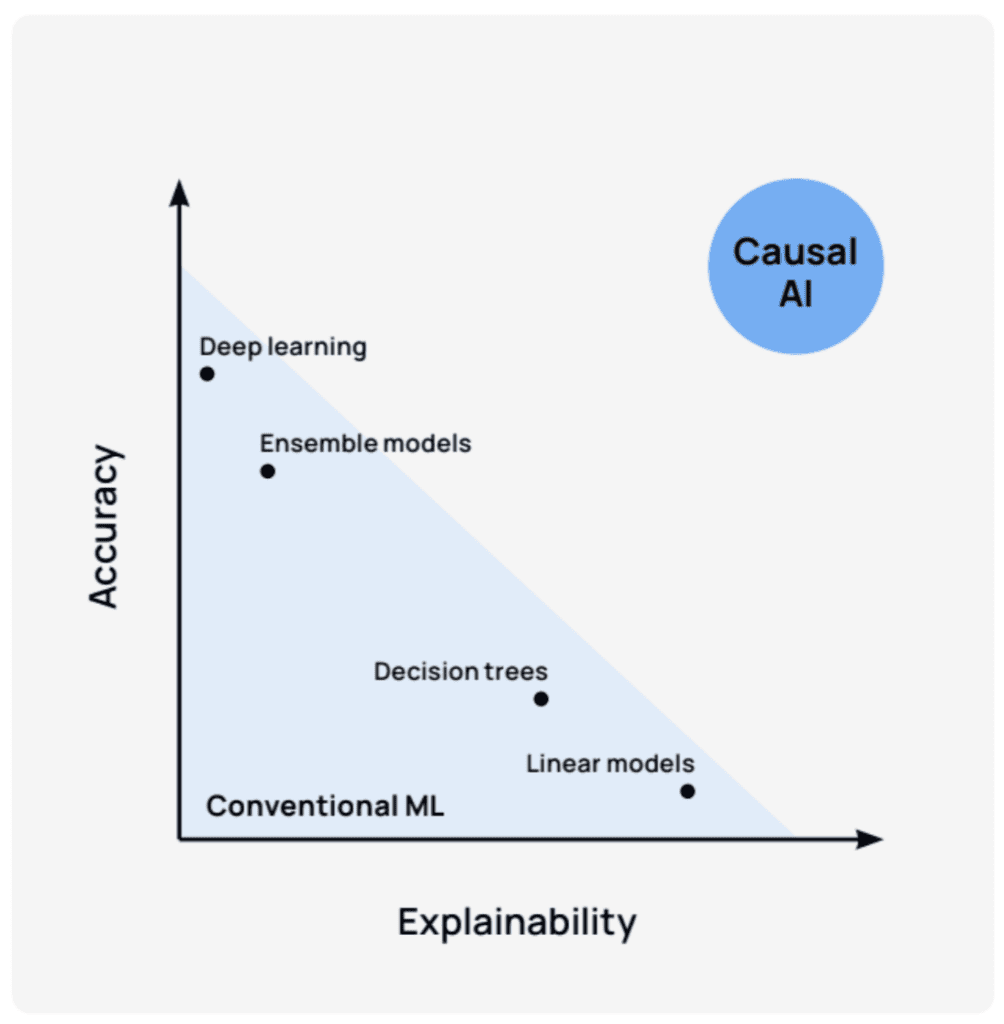
Finally, with conventional machine learning, there’s a trade-off between explainability and performance — more powerful models sacrifice explainability. In contrast, a better causal model explains the system more completely, which results in superior model performance. Please check out the causaLens blog to take a deeper dive.
How does this impact my business?
Causal AI is the only form of AI that meets the FEAT principles in letter and spirit.
Embedding causality, and hence FEAT, in your AI systems doesn’t just mitigate risks — it also radically improves your business’ chances of successfully scaling AI adoption.
Many AI experiments are bottlenecked due to regulatory reviews. Causal AI removes this bottleneck with faster AI governance, and thereby radically accelerates the process of getting models in production. Another blocker in the data science workflow is that the model-building process often excludes human judgment and expertise, and the assumptions behind models cannot be satisfactorily explained to humans. Causal AI unites business stakeholders and data scientists in the model-building process, ending the lengthy and frustrating iteration cycles between business and data teams.
In conclusion, we want to emphasize that there are many ways to go about embedding causality, and therefore FEAT, into your AI systems. One is to adopt Causal AI for model risk management and evaluation — leveraging causality to evaluate and certify the inherent risk in a productionized model. causaLens’ model risk evaluation application can:
- Check that an existing AI system is FEAT-compliant — including monitoring and eliminating AI bias, promoting external accountability, and boosting model explainability.
- Generating documented risk assessments that are compliant with SR 11-7 and other leading-edge regulations.
- Monitoring systems for early warning signals of abrupt regime shifts under which models will fail, and detecting whether models are picking up on spurious correlations that are liable to break down.
- Diagnosing why models are underperforming and generating shareable explanations of model performance that business stakeholders can understand.
Speak with one of our experts in banking, insurance, or asset management to learn more about how Causal AI can de-risk your AI systems and boost responsible AI adoption.