Empower Experts with Causal AI
Causality is central to how humans, both as individuals and collectively as businesses, think about and engage with the world. What motivates our customers? What if we increase prices? Why has that portfolio underperformed? All of these are causal questions. Causal AI, the next generation of enterprise AI technology, also sees the world as a causal system. Causality is a shared language that enables humans and AI to communicate directly, in ways that conventional machine learning (ML) doesn’t allow.
The result is a synergistic human-machine partnership —one important dimension of which is seamless knowledge
integration between human and machine. Users can share their rich knowledge of the world with the AI. The AI, meanwhile, augments expert knowledge and judgment with rapid, data-driven inference, and explains its findings to users in language they understand.
By empowering experts to integrate their insights with AI, an organization can solve high-complexity business challenges — challenges that are often beyond the limitations of either human-only or machine-only analysis.
Experts + Causal AI
While experts know their territory — a sector, customer segment or business function — inside out, they also have
human flaws such as limited processing capacity and potential blind spots. So when organizations have access to well-validated algorithms, they should consider using them. Causal AI gives businesses the option to use models
that optimize their processes without any human input at all, and it can often be valuable for the AI to work autonomously in this way.
By blending expert judgment with AI, businesses can optimise forecasts, processes & decisions
But expert judgment should not be wastefully brushed aside. As Philip Tetlock argues in his book Superforecasting, “we need to blend computer-based forecasting and subjective judgment in the future”. Just as we could do this for forecasting, we could also do so for business process optimization and decision-making. Causal AI fulfils this vision by opening many novel ways in which humans and machines can communicate. Here, we illustrate two.
Causal Diagrams: A Common Language
The simplest way in which experts can interact with Causal AI is by directly enhancing the AI’s understanding of a given causal system. They can do this via causal diagrams, which represent the machine’s knowledge of the cause-and-effect relationships that underlie the observable data — territory that ML models can’t normally access. Crucially, they can be understood at a glance by humans.
An expert can share granular, complex information about the relationships between causal drivers and their effects. This includes how causal relationships change over time and depend on broader market conditions.
The advantages of this interactive functionality are greatest when data is unavailable or poor quality. In these common situations, ML algorithms, which require mountains of data, are stuck, while humans can give Causal AI the information it needs to produce results.
Take a simple causal model of air cargo demand for routes from Taiwan to the UK. Causal drivers will include, among
others, measures of economic health, such as Taiwan’s GDP, the strength of the trading relationship betwee Taiwan and the UK, UK foreign direct investment into Taiwan, and more up-to-the-minute indicators, such as data on e-commerce orders placed in the UK for goods in key Taiwanese export categories.
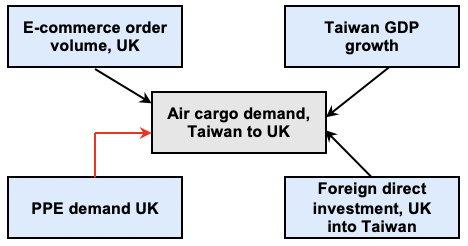
There may not be historical data on a relatively new phenomenon, such as the urgent need for PPE, produced mainly in Asia, which has created a demand surge for air cargo. An expert can directly convey to the AI how PPE demand impacts air cargo, and how this relationship will change over time and hinge on, say, production of medical supplies in Europe and coronavirus case numbers.
Causal AI autonomously adapts to crises faster than ML algorithms, but experts can turbocharge the process. Their knowledge can be used to find the right causal model even before data sets become available, giving organizations a head start on the competition.
Once the AI has an accurate causal model, the potential applications are vast — from vanilla forecasting to simulating business decisions.
Experts Enable AI Business Challenges to Solve Complex
A second way in which experts can collaborate with Causal AI is by equipping it with the context that’s needed to solve complex business problems.
Consider an international courier: they need to reliably transport goods to schedule over thousands of miles and to millions of destinations, meeting demanding customer expectations and unforgiving standards of operational precision.
Fleet optimization is a strategic necessity. This requires transport AI to intelligently anticipate demand rather than reacting to it. Using Causal AI, the courier can build adaptable, explainable supply-and-demand models, of the kind illustrated above, across all its routes.
With expert knowledge, Causal AI can solve business problems in the face of shifting markets, challenging operational constraints & severe uncertainty
The expert can then feed in the constraints needed to solve the business challenge. They can specify an optimal cargo load factor (say, 60%) to meet efficiency targets. And instruct the supply chain AI on how to group items: fragile items with high load complexity may need to be handled together, while perishable goods may require a cool supply chain. There may also be constraints on the number of aircraft that should be simultaneously in flight, for contingency planning.
With the benefit of expert-defined context, Causal AI can then optimise the courier’s air fleet, dynamically updating decisions on the basis of its predictive models, factoring in uncertainty and challenging operational constraints. Conventional ML systems have no scope for integrating expert-specified business context and so can’t even attempt to solve these kinds of optimisation problems.
Feature Selection is a Blunt Instrument
Companies that rely on conventional ML systems can’t make best use of their in-house knowledge — their AI systems can’t operate in small data environments or solve optimisation problems that require expert- specified context. ML algorithms do permit users to select features for the algorithm to learn from, but this is a crude mechanism for integrating human judgment.
Feature selection doesn’t allow users to relay information to the algorithm about how a given feature relates to the
target variable, let alone how that relationship evolves over time or is sensitive to broader market conditions. It also pertains to a single model, and so is not of much help with AI-enabled business optimization, which requires many connected models and overarching human-specified contexts.
Conventional ML algorithms are unsuited to handling real-world, qualitative context
More broadly, ML algorithms are unsuited to handling real-world context. In the sci-fi series The Hitchhiker’s Guide to the Galaxy, a supercomputer named Deep Thought is asked what the meaning of life is — after eons of calculation it answers, absurdly, with a number: “42”. Correlations, which are also single numbers, on which ML relies, are similarly unsuitable for capturing the expert’s knowledge of qualitative, contextual relationships.
Towards Human-Machine Symbiosis
Human-machine collaboration is so fruitful because AI systems complement human strengths: they are good at tasks we are bad at, and not subject to biases or groupthink. What differentiates human-like Causal AI as an especially effective teammate is that it not only complements human thinking, it understands it too.
With human experts in the loop, Causal AI is able to enhance an organisation’s knowledge, even when data isn’t yet in digital form, and help it to solve complex business challenges. But there are many more sides to the human-plus Causal AI symbiosis. We will spotlight in future articles how humans and AI can together climb the ladder of causality, exploring huge domains of inquiry that are beyond the reach of less intelligent conventional ML systems.