- Blog
Next Generation A/B Testing powered by Causal AI
Introduction
- Causal AI makes A/B tests and randomized controlled trials more efficient and more generative of rich insights.
- Causal AI replaces A/B tests when it’s infeasible to run an experiment

Experimentation is needed, but a challenge
Remember Digg? If you don’t, you’re not alone! Digg was once one of the leading online social networks, until a single misguided product release decimated their user base. Getting a product release right (and avoiding being a Digg) requires understanding the customer, and channeling that understanding into new features and offerings. And this requires experimentation, (spoiler alert!) or Causal AI.
As an example, let’s take an e-gaming company that causaLens has partnered with. They had a global product launch in March 2020, just as the world entered lockdown. Following the release and through to the end of 2021, revenue skyrocketed. As revenue subsequently collapsed in early 2022, the company came under pressure to understand why. And at a more practical level, they needed to figure out how to invest in and release version two of the product.
Some of the critical questions they faced included:
- Should we even invest in the release, period?
- What’s the expected impact of the release on revenue?
- If we do invest, which product features should be prioritized?
- How should we schedule the release?
Experimentation via a phased release can shed light on these questions, but that comes with big costs. Releasing v2 on a random subset of the market can undermine the community that’s emerged around the game, and also carries reputation risks for the company. How can the e-gaming company gather decision-critical insights with limited scope for experimenting?
Virtual Experimentation
Causal AI, a new category of machine intelligence that discovers and reasons about cause-and-effect relationships, can come to the rescue. Causal diagrams are at the heart of Causal AI. At an abstract level, these diagrams describe “what causes what” and how a system functions.

Back to our e-gaming example, the first problem the company is confronted with is answering the following counterfactual: what would have happened to revenue had we not released version one of the product in the first place? Lockdowns certainly were responsible for some revenue uplift for the e-gaming company — but how much? From a Causal AI perspective, we start by exploring this simple diagram:
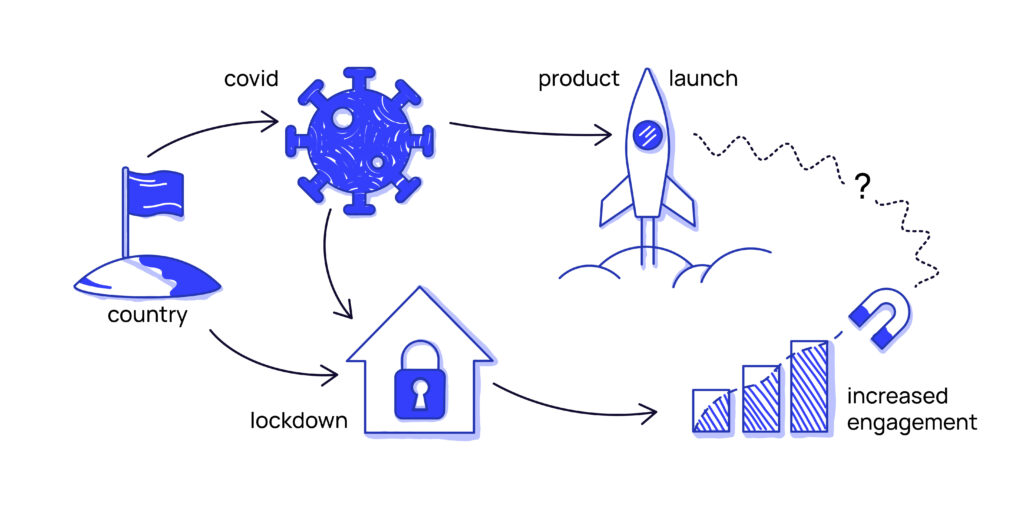
It’s of course not possible to wind the clock back and randomly assign some markets to a lockdown. The good news is that typically patterns emerge in the data that act as natural experiments — conditions under which nature randomly assigns otherwise similar customers to different treatments — gifting information for free without having to run an actual experiment.
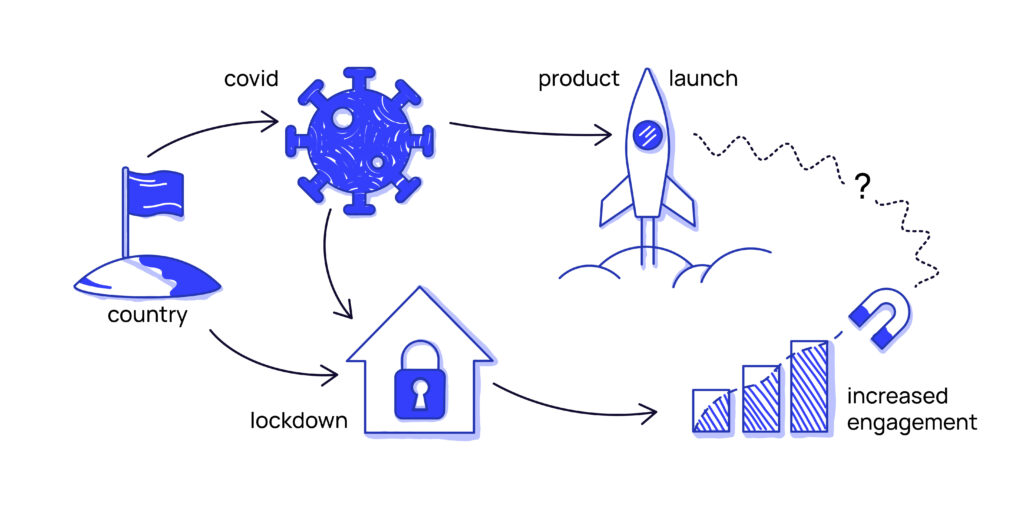
Different countries entered lockdown at different times and with varying levels of austerity. Although we don’t have worlds in which the lockdown didn’t happen, we have similar countries in which it happened differently enough, such that we can isolate the effect of the lockdown from the effect of product launch. In reality, by using external data we can quantify the strength of the lockdown for each different city at each point in time, adding a completely new dimension to the problem.
Natural experimentation with Causal AI is similar in approach to methodologies in Economics that were awarded the 2021 Nobel Prize. To give a flavor of this work, Nobel Laureate David Card investigated the effect of minimum wage on employment by comparing similar restaurants on the Pennsylvania-New Jersey border after the minimum wage in Jersey was raised from $4.25 to $5.05. (Contrary to conventional wisdom, he found that the negative effects of increasing the minimum wage are small).
Causal AI can emulate this logic. Returning again to our use case, Causal AI can zero in on countries with similar demographics but different lockdown policies, such as Sweden and Denmark, to tease out the causal impact of lockdown on engagement. While Sweden had lax policies, Denmark had far stricter isolation policies. If we find much higher revenue growth in Denmark than Sweden, it’s likely due to lockdowns — whereas if they’re both comparable, it’s likely due to the product release.
Next Generation Experimentation
Reality is naturally more complex. The customer base varies vastly between countries: from age, to disposable income, and even the amount of time users have available to interact with the product. This makes direct country-to-country comparisons challenging — two countries that look similar may be vastly different.
What if we could anticipate on a person-by-person level the impact of an intervention on their behavior — taking into account all of their personal characteristics and exogenous factors? Relying on A/B testing alone, this would be enormously resource intensive. It would require isolating the impact of age, income, levels of engagement, time of the day. And some experiments simply cannot be conducted: we cannot isolate exogenous factors, such as the state of the economy or the weather!
With Causal AI, the process becomes simpler. To turbocharge A/B tests, we start by looking at observational data and extracting initial causal insights, as described in the previous section. Using causal discovery from observational data it is possible to construct a causal graph relating every possible meaningful factor in the data , and understand how these factors will affect revenue.
In some cases, it’s just not possible to gather the needed insights from the data. In these cases, Causal AI requests domain expertise or recommends the exact A/B test that will resolve the ambiguity. Instead of running large-scale and expensive experiments, Causal AI alerts you to the factors that need testing and the right subsection of the population on which to run these tests. A/B tests can also be prioritized based on the potential revenue uplift from learning the new information.
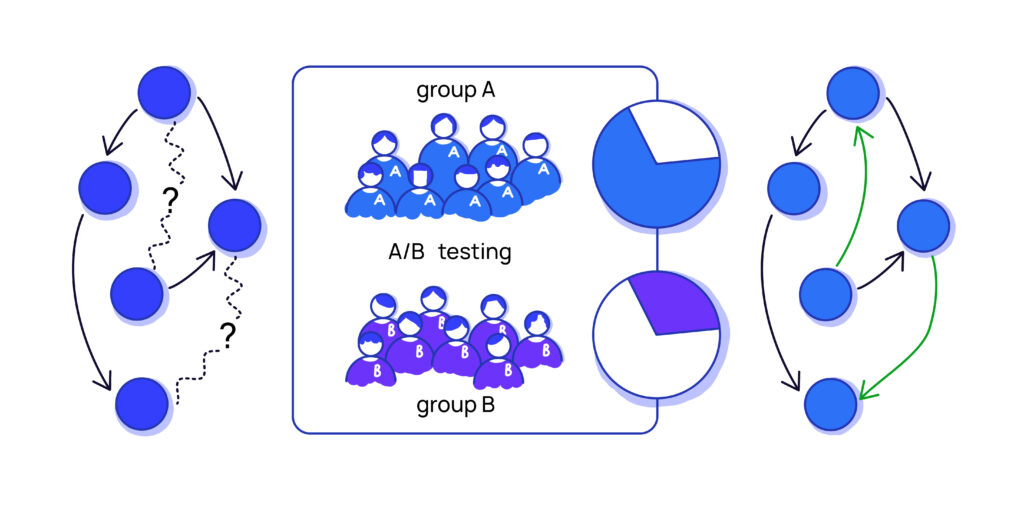
The ROI for the business is clear: instead of running large, monolithic A/B tests — we now have a live system that recommends, schedules and designs tests on a much smaller scale — making experimentation much cheaper, and introducing guardrails to ensure that there are no harmful effects downstream.
Conclusion
We’ve highlighted how Causal AI can step in when A/B testing is infeasible, and how it can enable businesses to squeeze richer insights out of the A/B tests they do run.
Our case study has focussed on product releases, but experimentation is fundamental for all customer-centric organizations. Set up a call with one of our consultants if you’d like to learn more about taking your A/B tests to the next level.
Originally posted on Medium, Turbocharge A/B testing with Causal AI