Customer Case Study: Client Retention
North American pension plan improved beneficiary satisfaction and increased retention by 17% using decisionOS powered by Causal AI.
North American Pension Plan
Financial Services (Retirement Plan)
Customer Satisfaction/ Retention
17% increase in retention
The Challenge
The pension plan manages money for millions of individual stakeholders, providing a range of accounts, funds and retirement services. Managing customer satisfaction and ensuring that they meet their customers’ financial needs is a key priority of the plan.
Following the death of a plan holder, funds may be passed to beneficiaries. These beneficiaries may keep this money with the plan or withdraw it. The pension fund identified that recipients of large settlements often withdrew these funds.
They wanted to understand how much the amount and type of customer interactions influenced retention.
Using standard machine learning approaches, it was difficult for the plan to understand the optimal strategies to improve retention.
-
1Relying on historical correlations
Historical correlations can be spurious leading to suboptimal retention strategies
-
2Explainability
Existing methods make it difficult to understand which touchpoints are driving retention
-
3Focus on predictions
Machine learning focusses on forecasting instead of simulating the effect of interventions (“what-if? questions”).
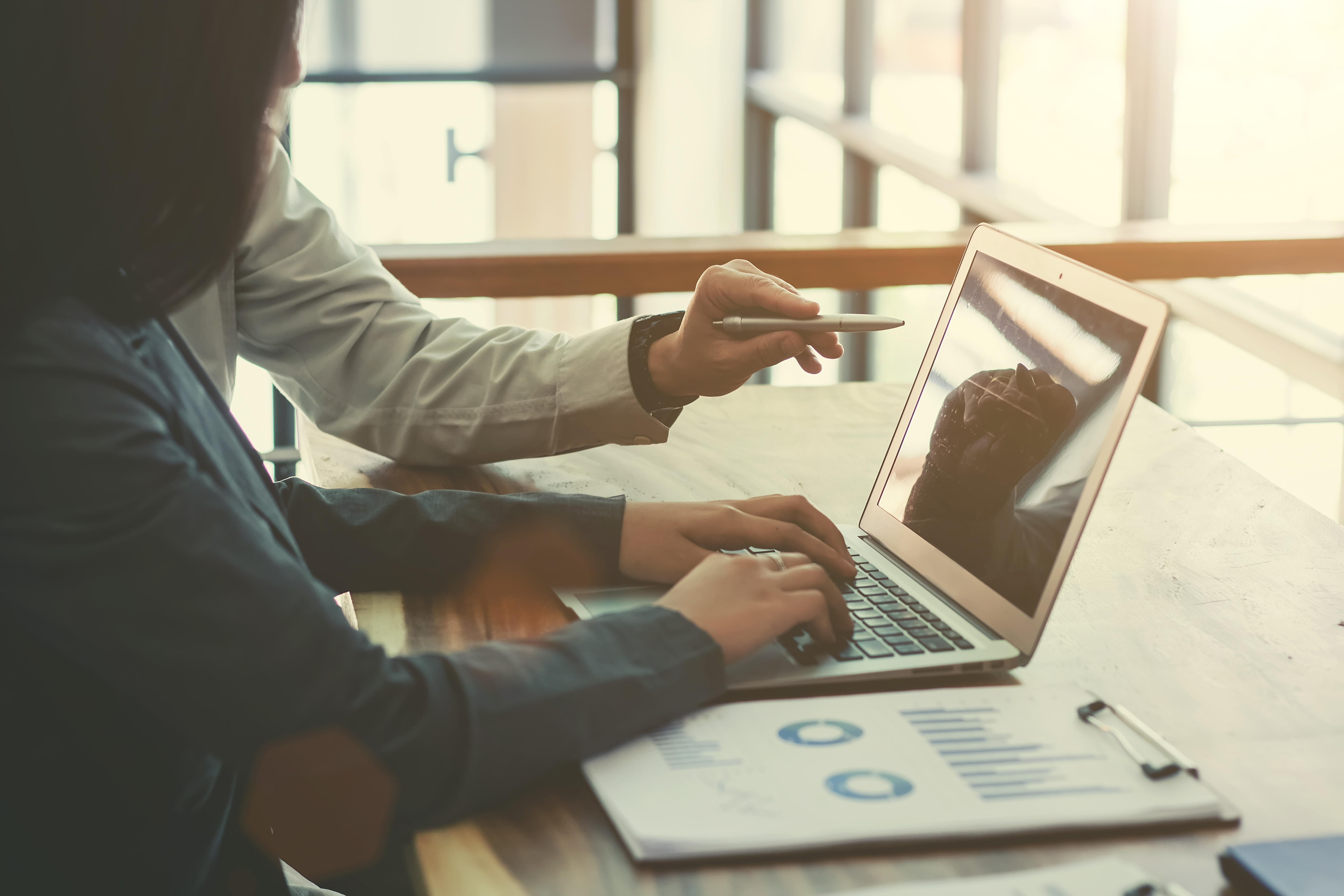
The solution
The plan turned to causaLens to build trusted retention models. Using decisionOS the client was able to:
- Identify the causal drivers of customer behavior, accounting for confounding variables such as the demographics of the beneficiary and the structure of the accounts
- Work together with the business stakeholders to build an explainable model that captures the complex interactions between these variables in a clear and transparent way
- Go beyond predictions leveraging counterfactual and interventional scenarios to create optimal retention strategies
- Build a decision app helped non-technical stakeholders understand the intuitive dynamics of the model and apply counterfactuals to help with decision making (for example: “what if all clients received our highest tier of servicing?”).
Results and Benefits
Through use of the model and the decisionApp, the plan was able to identify interventions that could be made to their servicing approach to improve retention by an estimated 17%.
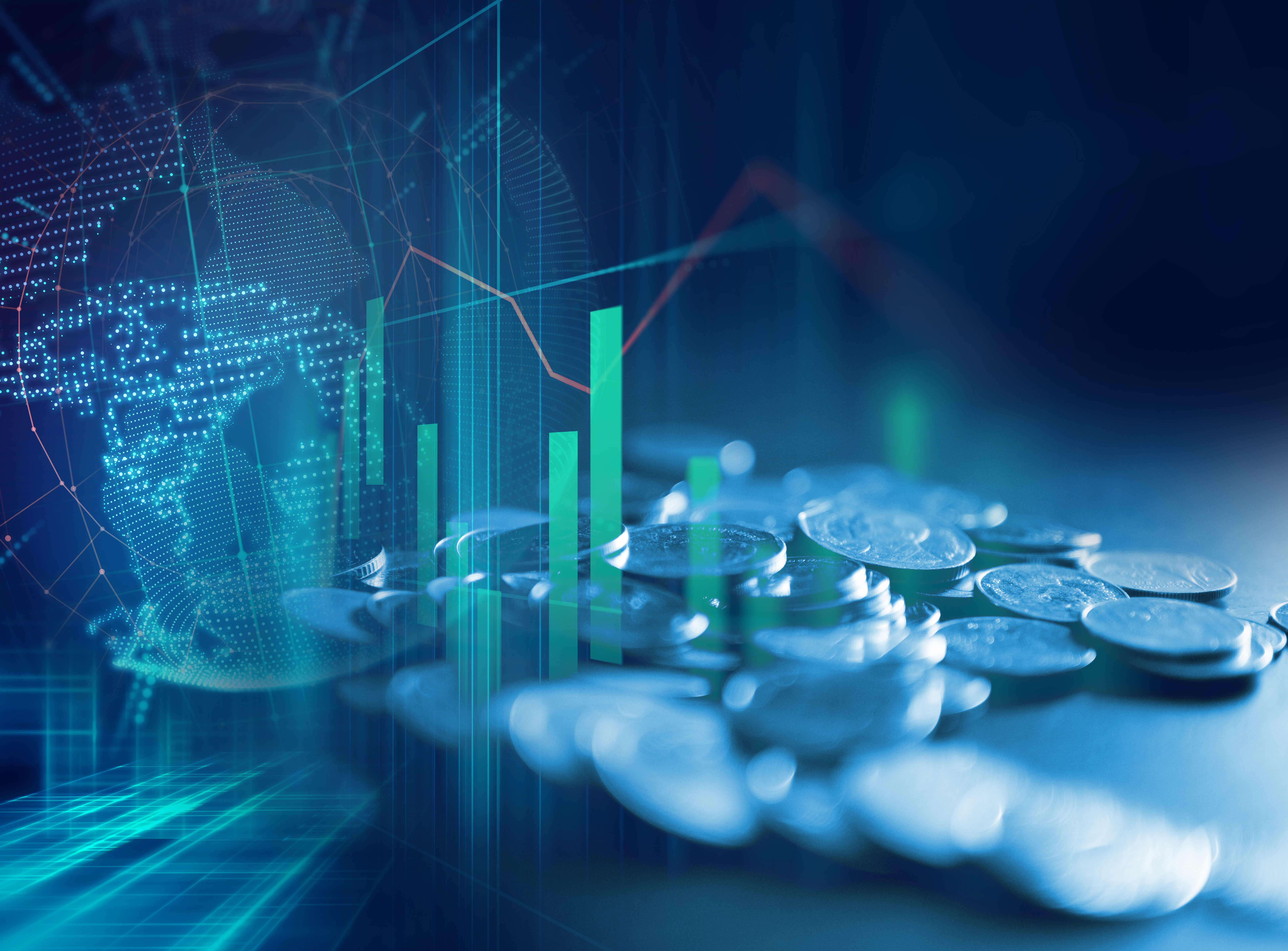