- Research Papers
Causal Analysis of the TOPCAT Trial: Spironolactone for Preserved Cardiac Function Heart Failure
23 November 2022, 17:22 GMT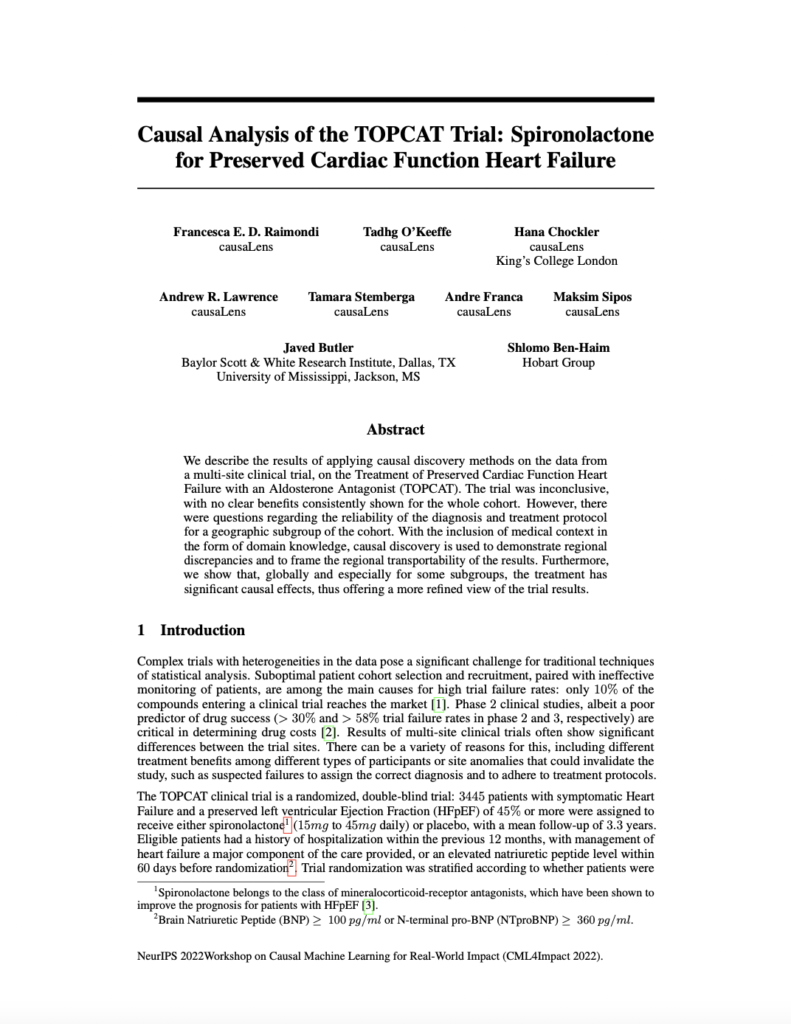
Our Analysis of the TOPCAT Trial:
Complex trials with heterogeneities in the data pose a significant challenge for traditional techniques of statistical analysis. Suboptimal patient cohort selection and recruitment, paired with ineffective monitoring of patients, are among the main causes for high trial failure rates: only a minority of the compounds entering a clinical trial reaches the market. Phase 2 clinical studies, albeit a poor predictor of drug success, are critical in determining drug costs.
Traditional methods based on correlation and survival analysis are prone to confounding and struggle to capture complex interactions: causal discovery is a necessary step in overcoming these issues. Causality can offer new insights into clinical trials: causal methods have shown promise for analyzing results from Randomized Controlled Trials (RCTs), via target trials, transportability, and prediction invariance.
This paper, presented at NeurIPS 2022 workshop Causal Machine Learning for Real-World Impact, shows that causal discovery allows us to understand the causal pathways of the main outcomes of the TOPCAT trial, by deriving a causal diagram that provides insight into the regional transportability of the trial results. We show that, globally and especially for some subgroups, the treatment has significant causal effects, thus offering a more refined view of the trial results. We were able to demonstrate regional differences of the causal mechanisms between the two main geographical subsets of the trial, offering evidence of the controversial discrepancy of the original study (Western vs Eastern countries). Finally we show that the identified causal models can be linked to a more traditional survival analysis of the trial data, achieving better explanatory performance.