5 Steps to Prepare for AI Regulations
AI regulations are arriving, and rapidly. Abstract frameworks and guidelines are crystallizing into binding regulations with big penalties for noncompliance. The ambitious European Approach to AI is a case in point — it will hit rulebreakers with fines of up to 6% of worldwide annual revenue.
Across all sectors of the economy and jurisdictions, regulators are converging on a common set of core AI governance best practices. The emerging consensus is that algorithms must be explainable, free from bias, robust, aligned with human values and objectives, and continuously monitored for risk.
“Rulebreakers will be hit with fines of up 6% of global annual revenue.”
The European Commission
AI leaders are proactively doubling down on mitigating regulatory-compliance risks. This is a win-win: enterprises that have strong AI governance not only reduce risks but are also far more likely to scale AI across the enterprise.
Causal AI, a new kind of machine intelligence that mirrors human reasoning styles, enables enterprises to meet the most demanding AI governance requirements while scaling AI adoption. We set out 5 steps you can take with Causal AI to prepare for the new regulatory landscape.
1
Build AI systems that are genuinely explainable
Explainability is a fundamental aspect of nearly all AI regulatory proposals. It’s critical that data subjects can understand and challenge algorithmic decisions that impact them. And enterprises need to understand their models in order to de-risk and trust them.
Causal AI models are intrinsically explainable — unlike complex machine learning systems, they don’t require additional, external models to reveal their assumptions. Anyone can comprehend the assumptions they’re making. Learn more about how Causal AI produces superior explanations here.
Singapore’s cutting-edge “FEAT”. (Fairness, Ethics, Accountability, and Transparency) guidelines assert that higher-risk AI systems should be intuitively explainable and provide actionable insights. The Singaporean regulator notes that the best explanations “appeal to causal structure rather than correlations”. |
The best machine explanations appeal to causal structure rather than correlations.
The Monetary Authority of Singapore
2
Ensure algorithms are free from AI bias
AI has a bias problem, and regulators are clamping down on it. Infamous examples of AI bias include sexist HR tools, discriminatory credit-scoring applications, and racist facial recognition software. The problem is that correlation-based machine learning algorithms project biases that are implicit in historical data into the future.
Causal AI is the only technology that can guarantee that ethically sensitive information is not inappropriately impacting algorithmic decision-making. Find out how Causal AI solutions can plug into your ecosystem and certify that your AI systems are fair.
In the most recent development in the regulation of AI (May 26, 2022), the US Consumer Financial Protection Bureau has insisted that antidiscimination laws must be extended to decisions taken by algorithms. It’s the most recent call for AI bias regulation — but it won’t be the last. |
3
Prevent overfitting, promote robustness
Correlation-based machine learning, especially more powerful ensemble models and neural networks, have a tendency to “overfit”. In other words, they memorize random noise in data rather than learning meaningful patterns. This leads machine learning models to break down in production in the real world when conditions change (think COVID-19).
Regulators are calling for more robust AI. Causal AI promotes robustness by ignoring noise and zeroing in on a model that represents the true drivers of a given system.
The European Parliament has issued recommendations for the financial sector, following up on the Commission’s proposals. They highlight that models often fail “when novel market conditions differ significantly from those observed in the past”, and emphasize the need for more robust systems. |
4
Align algorithms with human intuition and objectives
Like oil and water, humans and conventional machine learning algorithms do not tend
to mix. Machine learning models are built without much in the way of human input, beyond maybe selecting features to include in the model. This adds layers of risk to AI systems and leads to unexpected failure modes.
Many regulators demand that higher-risk AI systems are overseeable by humans and involve some element of human-in-the-loop control. Causal AI enables domain experts and data scientists to work collaboratively with machines to build less risky and more human-compatible models.
Take a deeper dive into Human-Guided Causal Discovery in our webinar.
The European Commission’s watershed draft regulations demand that higher-risk AI systems involve “human oversight throughout the AI systems’ lifecycle” and should be developed “with appropriate human- machine interface tools”. |
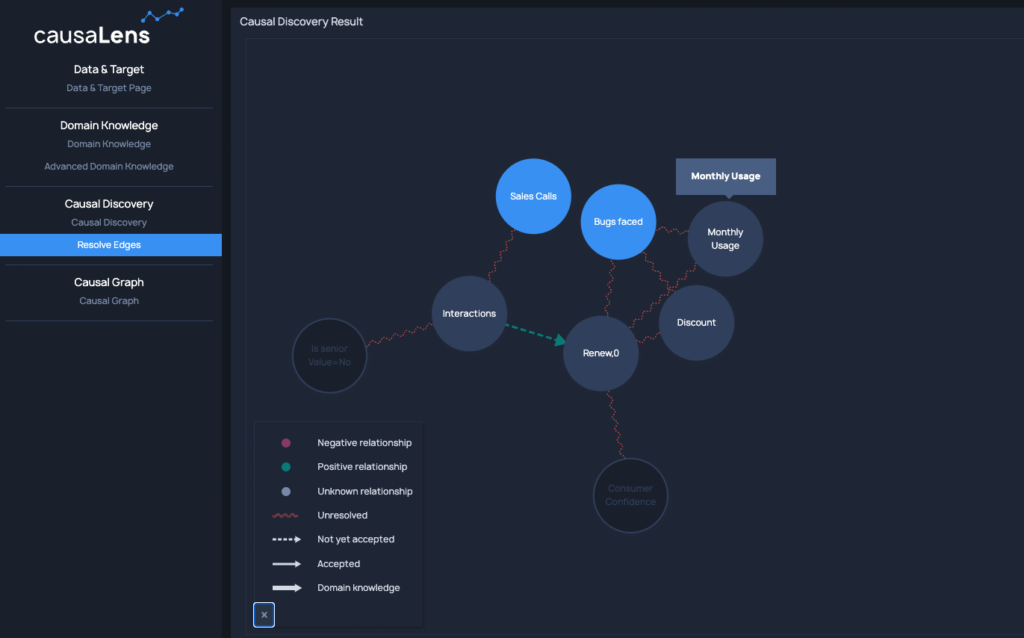
5
Continuously and proactively monitor for risks
AI governance doesn’t end when you’ve productionized a model. Models should be continuously monitored — to ensure that they’re adapting to real-world dynamics and are remaining free from bias. Beyond monitoring, you need to proactively prevent model failures. And if models get stuck you’ll need advanced model diagnostics to get them unstuck.
causalOps is a Causal AI-enabled risk monitoring tool that does just this. causalOps finds root causes that uncover why models are failing, it raises early warnings of “regime shifts” (basically, significant changes in the world), and runs scenario analyses to check how models will perform in different regimes. These capabilities aren’t available with vanilla MLOps.
Think of AI systems for fraud prevention. Is the machine failing due to a novel fraud tactic? Is the model itself an attack surface? How will changes in the macroeconomic environment impact fraud rates, and how will the model cope? causalOps resolves these questions in-flight to de-risk productionized models.
In the Commission’s influential draft regulations, model risk- management and postmarket monitoring are central requirements on organizations using high-risk AI systems. Many other regulators are following the EU’s lead on risk monitoring. |