AI that speaks the Language of Executives and is aligned to their Objectives
Talking Points
- The job of the executive has suddenly become even more challenging as various stakeholders demand that they do not fall behind in the AI race.
- Traditional AI techniques & Generative AI are applicable for certain use cases, especially around unstructured data & creative tasks, but do not offer promise for improving decision making in the enterprise.
- According to the definitions of Judea Pearl’s ladder of causality, most enterprises are currently stuck on level 1, “Association”.
“Association” often helps with predictive insights but that’s often not enough.
Climbing up to the levels 2 and 3, “Intervention” and “Counterfactuals”, unlock the true power of AI for the enterprise. - Causal AI helps executives bring their organizations to levels 2 & 3, making it a must have technology.
Causal AI speaks the language of execs, through cause-effect relationships, not just statistical patterns, and is aligned to their objectives, driving actionable insights that improve business KPIs. - Causal AI has been featured in 3 Gartner hype cycles, including AI and Emerging Technologies.
causaLens has been named a cool vendor by Gartner. - causaLens is the pioneer of Causal AI, helping enterprises deploy & scale Causal AI across the organisation in the most efficient way.
The challenge for Executives in the age of AI
The recent AI inflection point has added another area of complexity for executives at Fortune 500s. Customers, employees and shareholders are all demanding clear AI strategies to ensure that companies remain competitive and are continuing to innovate. At the same time, executives need to be able to cut through the hype and find technologies which allow them to solve real business challenges which drive significant value. There is a need for urgency, but technology choices must be considered carefully.
This article will outline why Causal AI is a tool that both speaks the language of executives and is aligned with the objectives of executives. So for areas that executives care about, it promises to deliver significant value. Finally, it will outline why decisionOS by causaLens is the best way to build and scale this capability to quickly deliver value across the organization.
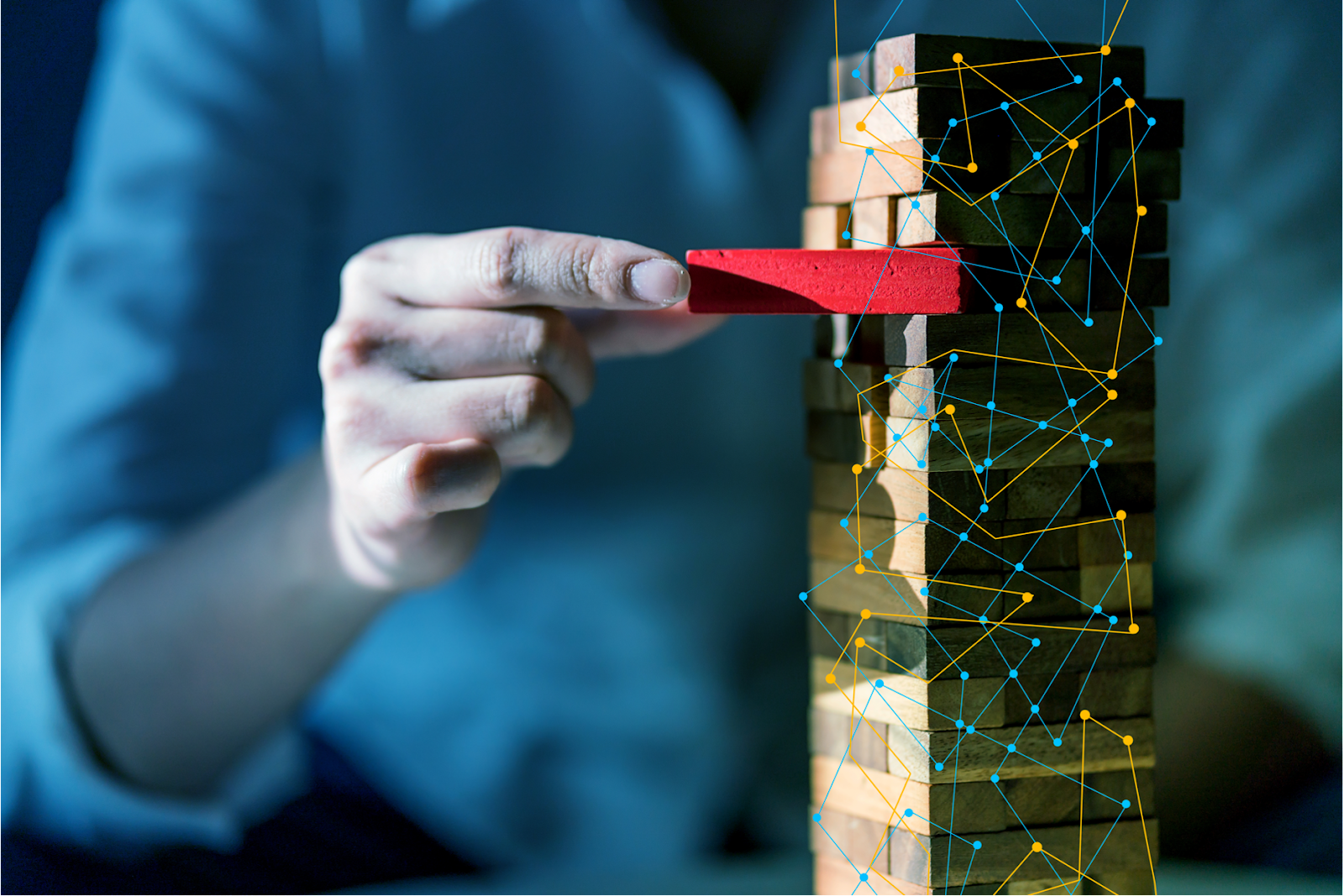
AI that speaks the Language of Executives
For AI to offer true business impact, solutions need to speak the same language as executives so that they are comfortable introducing it into the organization. Execs need:
- AI that is trustworthy and explainable, limiting business risk
- AI that is fair, ethical and human-centered
- AI that delivers clear, measurable value
Reduce business risk by using AI that understands cause and effect, rather than relying on complicated statistical patterns
For AI to become a mission critical part of the business, there needs to be an understanding of how it is being used to support decision-making and therefore what risk it carries. Traditional AI techniques, with their black box nature and basis in statistical associations, do not offer this type of understanding and so often end up being applied to less important or ‘nice to have’ use cases and can also provide disastrous results.
With Causal AI, all models are based on a fully explainable causal graph which tells the story of the business situation. Execs can quickly understand why a particular recommendation has been made and therefore have complete oversight when AI-based decisions are implemented. Overall, the level of AI risk within the organization can be easily controlled.
Read more on Explainable AI (XAI) doesn’t explain enough.
Ensure AI is fair, ethical and human-centered
One of the main challenges of the AI era will be ensuring AI is fair and human-centered. The capacity for AI solutions to go wrong is well documented with some high profile cases from large enterprises. Often, the root cause of this is taking a pure data-driven approach that does not consider key domain-knowledge, that may not live within the data. This results in a model that does not reflect reality, is very difficult to explain and ultimately offers poor recommendations.
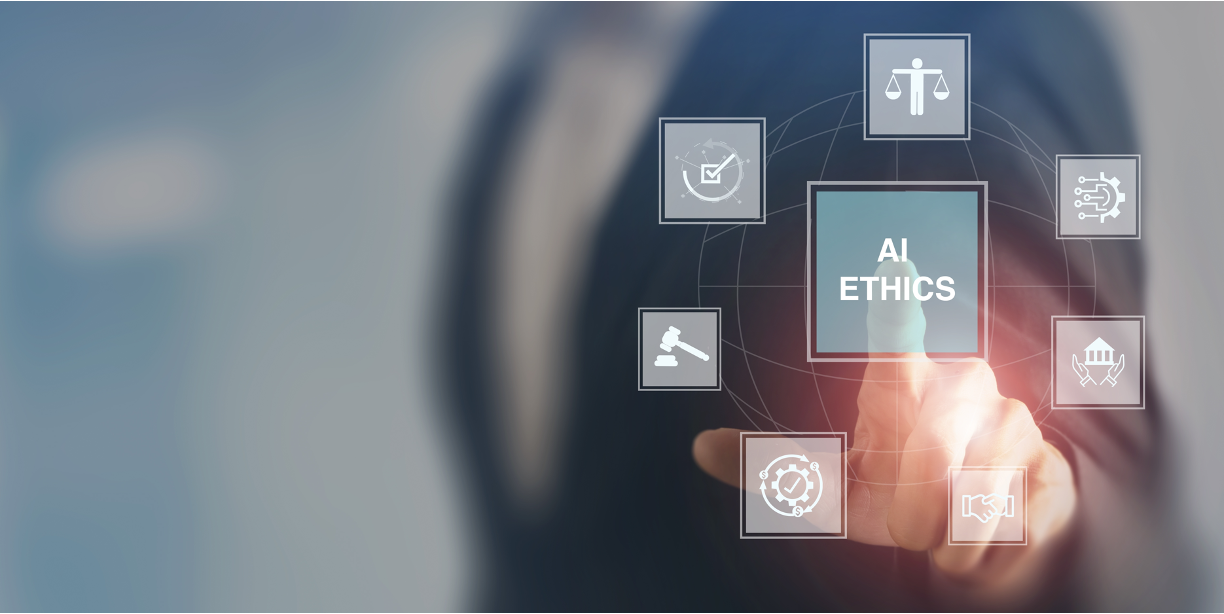
A better approach is to build a model that combines the hard-earned knowledge which lives within the organization with algorithmic techniques using enterprise data (read more on our Human Guided Causal Discovery framework). Causal AI models naturally provide this combination ensuring models accurately reflect the constraints of the business situation. This helps companies to avoid some of the painful results such as making decisions based on gender bias and leads to AI that is fair and human-centered.
Read more on AI fairness and causality
Read more on our fairness decision intelligence engine
Observe and track the value of AI deployments – Go beyond predictions with Causal AI, making better decisions and tracking decision success
One of the big challenges executives need to grapple with when it comes to AI is how to measure the value that AI brings to the business.
Traditional ML techniques are typically focussed on making predictions. But accurate predictions are not enough to improve decision-making. For example, consider a simple customer retention use case. Accurately predicting a given client is likely to churn is an interesting insight, but it does not inform the organization about what actions can be taken to prevent this client from churning. It may be the case that this particular customer is going to churn regardless of any interventions, so trying to prevent them from churning is a waste of resources that could be better spent elsewhere.
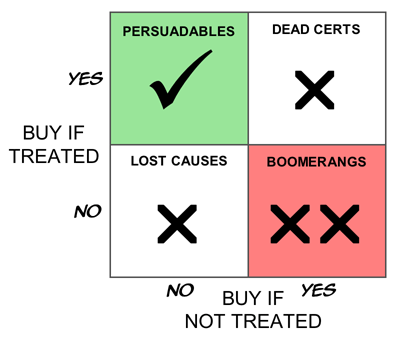
For a different customer, it may be important to know that they are not particularly price sensitive but that high service quality matters to them. So intervening with a discount is unlikely to help retain them. Predictive models are unable to capture these types of insights related to taking actions.
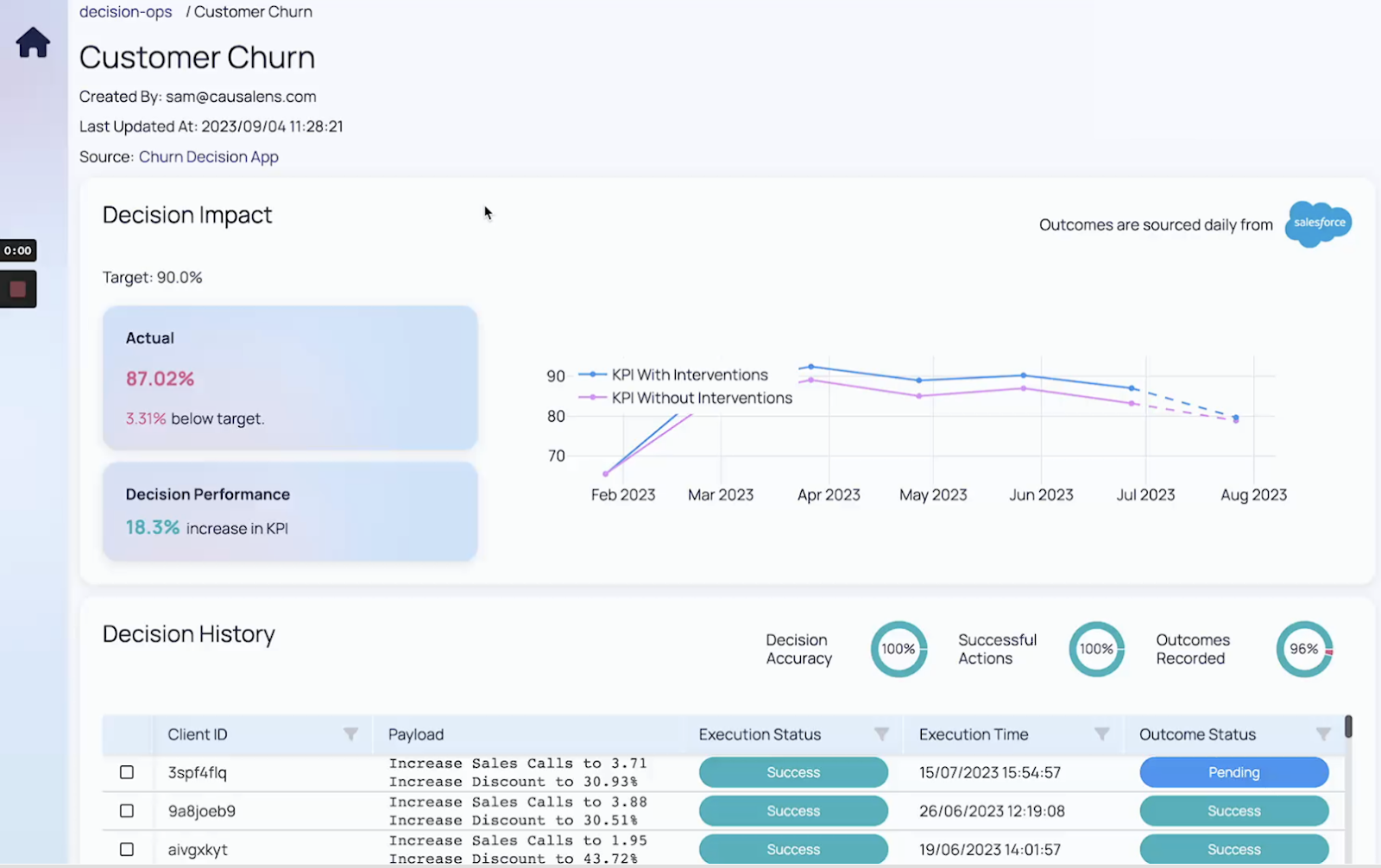
Additionally, even if an action is taken based on a prediction made by a model, traditional models do not typically contain a mechanism for measuring the effect that the intervention had. This results in little feedback on the success of a decision. With Causal models, recommendations are at the decision level e.g. offer customer X a discount of 20%. Following this, the causal model can continue to track the success of the decision. causaLens have productized the capability of accurately tracking the success of decisions within decisionOS.
Read more on decisionOps here + watch the presentation from our launch event
AI that is aligned to the Objectives of Executives
Causal AI solutions are successful as they align with the objectives of executives they naturally capture the effect on business KPIs and therefore improve decision-making:
- Causal AI is able to learn from the past by understanding ‘why’ previous decisions were made and why KPIs behaved in a certain way as a result.
We achieve this by mapping out the cause-effect relationships of the business KPIs using our Human Guided Causal Discovery framework - Causal AI can model future outcomes, improving decision-making.
We achieve this by building causal models and leveraging decision intelligence engines. - Causal AI model performance is tracked based on changes in KPIs rather than traditional data science metrics.
We achieve this with decisionOps, our framework for deploying, monitoring & measuring the impact of decision workflows
Learn from the past and understand why
Traditional ML techniques rely on statistical associations to make predictions. This can mean that they predict well. However, because they are based on statistical associations and not an understanding of cause and effect, they are unable to provide the ‘why’ behind a prediction. This is disconcerting for decision makers and is often the reason that a large number of data science models do not make it into production – they do not engender trust.
Every decision recommendation made using Causal AI has a clear ‘why’ behind it due to the fact that Causal models are always based on an underlying causal graph. Executives can easily inspect models, review the decision process and understand the effect on KPIs.
Connect the why to actions – make better decision today
Execs need to understand the effect or outcomes of different options they have when making decisions. Traditional ML models are unable to compute ‘interventions’ or ‘counterfactuals’, meaning they cannot provide an understanding of alternative options. The reason for this is that traditional models learn the underlying data distribution and then predict based on this. But intervening on a model necessarily involves moving away from this underlying distribution, so the ability to understand the effect of an action is impaired.
Computing interventions and counterfactuals is absolutely key when using AI to make decisions, this is why causality is a must for executives.
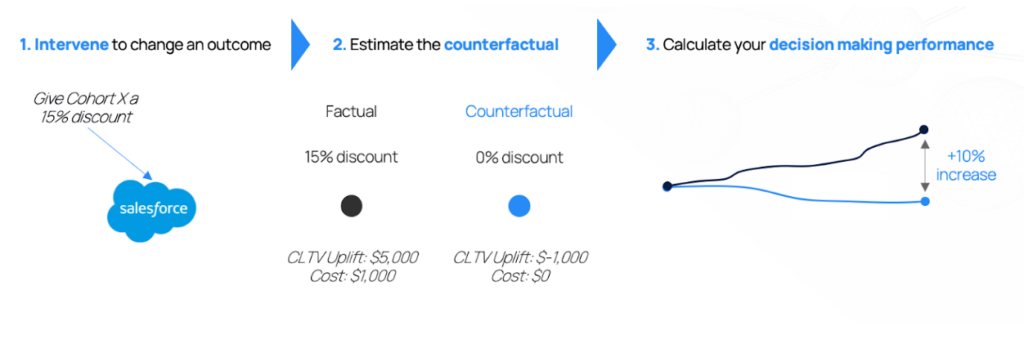
Understand impact at scale – Focus on business KPIs to drive the business forward
In order for Executives to derive value from AI solutions, solutions need to have a laser-like focus on improving business KPIs. Traditional ML and MLOps infrastructure has been built for predictive models in production, often monitoring model health using technical metrics. It is very hard to translate these metrics into actionable decisions. For example, often a ML model will be assessed on metrics like Accuracy and Precision but ‘good’ performance by these metrics does not necessarily translate into improvement in business KPIs. This is often where the connection between business stakeholders and data scientists is lost.
In contrast, the output from a Causal AI is naturally at the business KPI layer. So model outputs are assessed in terms of business decisions e.g. a next best action is recommended and the success of this in the real world (e.g. revenue uplift) is tracked. This is a completely different way of thinking about making and tracking decisions within the enterprise and can all be achieved with a causal model. causaLens calls this decisionOps, a powerful new capability that executives and business decision makers are using.
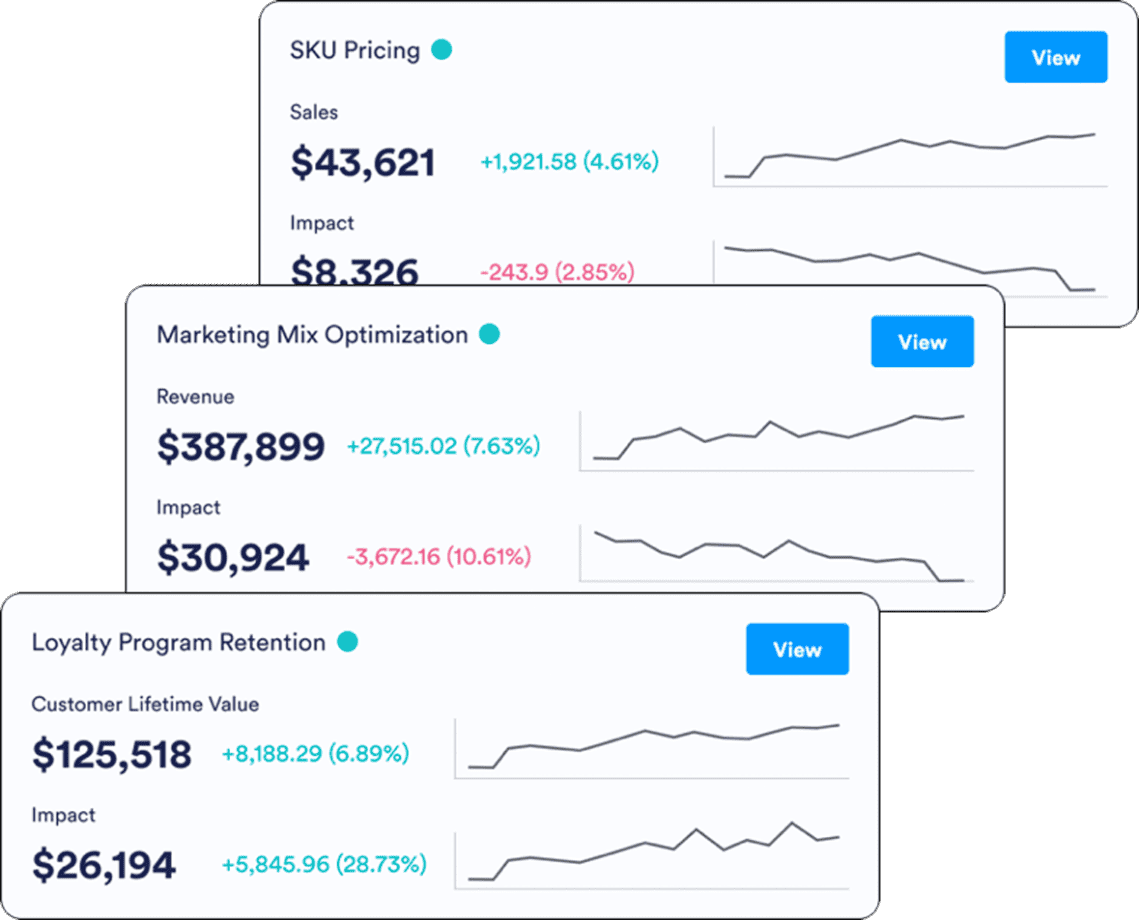
Climbing up the Ladder of Causality unlocks the true power of AI for Executives
The “Ladder of Causality” was proposed by pioneering researcher Judea Pearl.
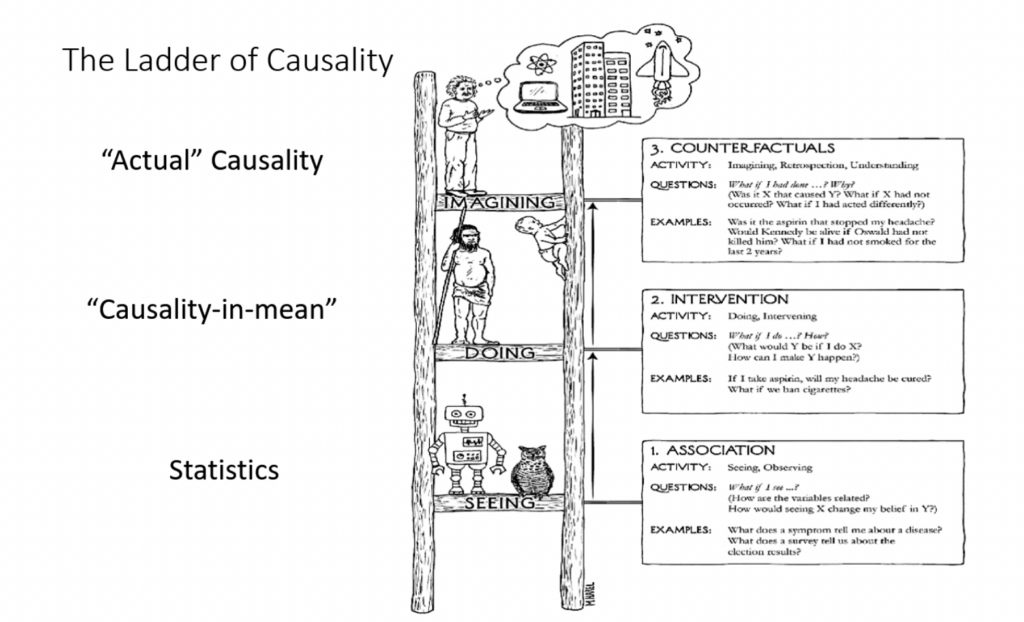
Current AI approaches currently only help with level 1, Associations – observing statistical patterns/association in data.
Black-box models are often deployed to help with associations introducing two main risks:
- They often confuse correlation for causation leading to non-sensical models and insights. Some examples are here.
- Their black-box nature leads to lack of trust from executives & regulators
Causal AI addresses these issues on the “Association” level.
However, Executives’ thinking & objectives aligns with Levels 2 and 3:
- Intervention: What are the actions we should take to improve our KPIs
- Counterfactuals: Imagining & assessing different scenarios to mitigate risks.
Causal AI helps executives reach Level 2 and 3, unlocking the true power of AI and actually aligning their objectives with those of the AI systems (read more below)
Enable the organization with this capability through the partnership with causaLens
causaLens has developed decisionOS – the only end-to-end Causal AI platform – with the goal of allowing executives to turn their data into better decisions at scale. decisionOS offers an enterprise experience to data science teams allowing them to build causal graphs and models, seamlessly collaborate with domain experts, and package up their work into decision applications which speak clearly to executives.
Read more here about why causaLens compared to alternative ways of implementing Causal AI.
The future of Enterprise AI
Causal AI is a new type of AI that is aligned to the objectives of executives and speaks the language of executives. Companies which utilize this powerful combination will be the ones that extract significant value from the fast growth in AI over the next 5-10 years. To date, causaLens has helped business leaders to make better decisions across a range of verticals including retail, consumer goods, manufacturing, financial services and many more.
Causal AI & Gartner
Gartner continues to recognise Causal AI as key technology in a number of areas by including Causal AI in its:
- 2023 Hype Cycle for Emerging Technologies
- 2023 Hype Cycle for Artificial Intelligence
- 2023 Hype Cycle for Emerging Technologies in Finance
Browse our case studies to learn about specific solutions we have deployed across use cases
We have brought to market the first operating system for decision making, powered by Causal AI – it’s enterprise ready and exponentially adopted by Fortune 1000s