How Human-Machine Teams Can Out-Think Fraudsters
At a Glance
Social engineering scams have exploded in recent years and pose acute challenges for banks, whose fraud defenses are continually out-maneuvered by changing attack strategies.
Causal AI, a new form of human-centered AI, gives banks an extra layer of intelligence in the fight against payment scams and novel fraud tactics. causaLens’ decisionOS:
- Anticipates and adapts to the evolving fraud landscape, by leveraging algorithms that go beyond memorizing old attack patterns
- Provides enhanced situational awareness, with tools to scenario plan and stress test emerging threats and intervention strategies
- Harnesses human intelligence and the best of the banks’ pre-existing solutions, bringing both within an AI control system
- Meets new EU regulations and supports cost-efficient complaint resolution via next-generation explainable AI
Banks that adopt causaLens’ decisionOS can expect to accelerate battle-ready model development by 10-30X and reduce the total burden of fraud by roughly 70%.
The evolving fraud landscape
Fraudsters and banks are competing in a high-stakes game of strategy that’s costing banks around 5% of their revenue every year. Fraud gangs are constantly developing new techniques to out-maneuver banks. One such is Authorized Push Payment (“APP”) scams — in which the customer is duped into authorizing a payment to an account controlled by a fraudster (see Fig. 1).
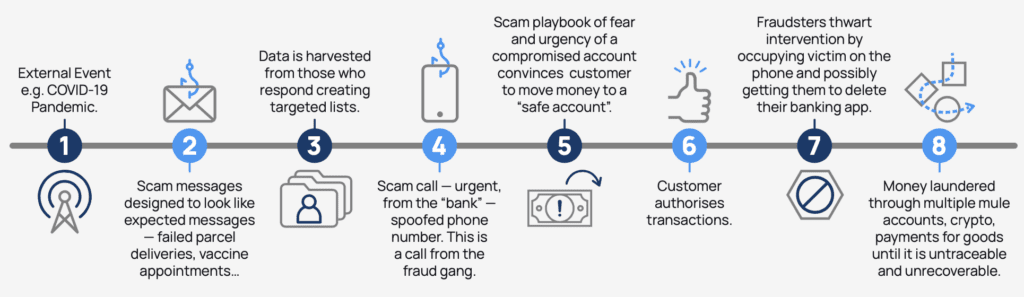
Figure 1. APP scams exploded during the pandemic, as fraudsters preyed on customers who were expecting vaccinations or home deliveries. Once embroiled in the social engineering process, victims are duped into believing that the bank’s interventions are part of the attack — they are convinced they have just stopped a fraud when in fact they have facilitated one.
APP scams have become one of the top challenges facing the industry globally, with the UK’s main banking body declaring them a “national security threat”, and with nearly 1 in 2 banks in Asia-Pacific naming them as their biggest problem. APP scams are particularly challenging for banks because ultimately the customer is authorizing the transaction, and so the bank’s normal controls do not apply.
Operational challenges in fraud prevention
The current fraud defense environment is a patchwork of solutions that can leave banks vulnerable to APP scams and other novel fraud techniques. These include rules-based and machine learning systems
Rules-based systems
Rules are easy to explain and are regulator-friendly. Unfortunately they are inaccurate, inflexible, and difficult for operators to manage. They also likely have a limited view of the data.
Machine learning
Nearly all banks are experimenting with machine learning (ML) for fraud prevention, which can analyze vast amounts of data in real-time. However, very few banks trust the technology sufficiently to seriously use it. Current ML technology faces some fundamental challenges:
- ML algorithms overfit to yesterday’s fraud tactics, leaving banks vulnerable to today’s and tomorrow’s attack strategies. The ML model itself can become an attack surface.
- ML solutions are often “black boxes” that are hard to explain and have surprising vulnerabilities. This makes them impossible to debug, improve, regulate, and trust.
- The ML workflow sees data scientists and business stakeholders take turns to (a) build models and (b) review their suitability in light of human judgment and expertise. Decision-makers and fraud operators cannot easily probe or query the models, resulting in long delays between iteration cycles.
As a result, fraud losses continue to rise steeply even as AI implementation has increased (Fig. 3). COVID-19 has been an accelerant of intensive investment in AI fraud defense but at the same time financial loss due to APP scams has spiked.
Figure 2. Payment fraud losses have risen steeply over the same period that anti-fraud AI software has been widely adopted. The attack surface has expanded radically due to digitization and the rise of online banking. Existing solutions have prevented runaway losses but are coming under increasing pressure. Data: Merchant Savvy.
Regulatory heat
Regulators expect banks to protect customers from authorized payment frauds. The EU’s Payment Services Directive (PSD2) requires that banks deploy software solutions to prevent payment fraud. Incoming regulations are set to enforce reimbursements from APP scams — something that most retail banks are already voluntarily committed to anyway.
At the same time, customers expect frictionless banking and satisfactory complaint resolution, which is an escalating cost for banks. In sum, banks need to get fraud interventions right, and they need to justify them to customers and regulators alike.
The Causal Fraud Decision System
A fresh approach to fraud prevention requires new technology — the Causal Fraud Decision System is that technology.
Powered by Causal AI
We are all familiar with the expression “correlation does not imply causation” (Fig. 4). Many of the shortcomings of standard machine learning systems stem from the fact that they cannot distinguish correlation from causation.
Figure 3. Correlation is not causation. Current machine learning systems often make nonsensical recommendations based on misleading correlations.
Causal AI is the only form of AI capable of distinguishing correlation from causation. Causal graphs are at the heart of Causal AI (Fig. 5). Let’s take an example. Naively looking at correlations (at least early on in the pandemic) might have led you to wrongly conclude that getting a COVID-19 vaccination leads to hospitalization. The true causal graph for this system reveals that age is a common cause of vaccination status and hospitalization, which drives the observable correlation. Getting this wrong results in the wrong conclusions about vaccine efficacy.
Figure 4. An example of a causal graph for the relationship between vaccination and hospitalization with COVID-19. Age is a common cause of both vaccination status and hospitalization.
Causal knowledge gives machines higher reasoning and decision-making abilities, that include anticipating the effects of plans and imagining what-if scenarios. These humanlike reasoning abilities enable Causal AI to integrate more closely with human users, who can shape and refine models based on their intuition and expertise. This reimagining of the AI workflow ends the iteration cycles that routinely delay and derail AI projects.
The Causal Fraud Decision System gives banks the edge over fraudsters:
- Control black-box models and other point solutions
We recommend a modular approach to fraud detection, in which existing solutions can be added to a causal graph (Fig. 7). This includes embedding black-box models within the causal framework. Causal AI compensates for the biases of these point solutions while harnessing them in its decision-making process.
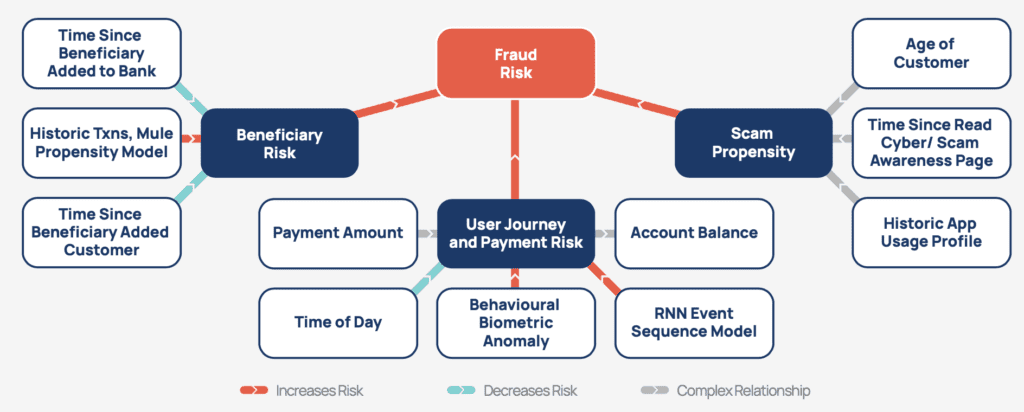
Figure 5. A simple example of an explainable Causal AI model for fraud detection. APP scam fraud risk is a function of the scam propensity of the payer, the riskiness of the payee, and the risk associated with the user journey. The Causal AI model can embed black-box machine learning algorithms that excel in identifying anomalous behavioral patterns that humans cannot detect (e.g. behavioral biometric anomalies), while maintaining overall explainability.
- Bridge the chasm between data scientists and operational teams
Operators can probe why a transaction has been flagged as fraudulent, and which customers and beneficiaries are high risk (“local explainability”), enabling swift complaint resolution. And senior decision-makers can examine and refine the assumptions behind the model (“global explainability”). Enhanced explainable AI tooling enables operational teams to scenario plan for emerging threats and explore the highest-ROI interventions for combating fraud (Fig. 6).
Figure 6. Users can run scenario analyses to zero in on the most effective interventions to combat fraud, and to explore the responsiveness of the model to new fraud strategies. In this example the operational team are exploring the effect of increasing the prominence of cyber scam awareness messages in the banking app and the projected impact on APP scams.
- Turbocharge model development and deployment
An intuitive human-machine interface and human-centered AI helps to radically accelerate building and productionizing models. Operators can supply operational constraints, guidance, and expertise, early in the AI workflow. Models are “battle ready” quickly and pass through governance checks easily as they meet all of the evolving regulatory requirements on transparency and trust.
- Anticipate the evolution of new fraud strategies
Powerful causal discovery algorithms combined with human intuition helps to guide models to ignore the noise and zero in on the variables that are really indicative of attacks (Fig. 7). Operators can anticipate the evolution of APP scams and other fraud techniques by exploring changes to variables that fraudsters can easily adjust, enabling teams to rapidly retool to meet emerging threats. The result is more resilient and adaptable fraud detection.
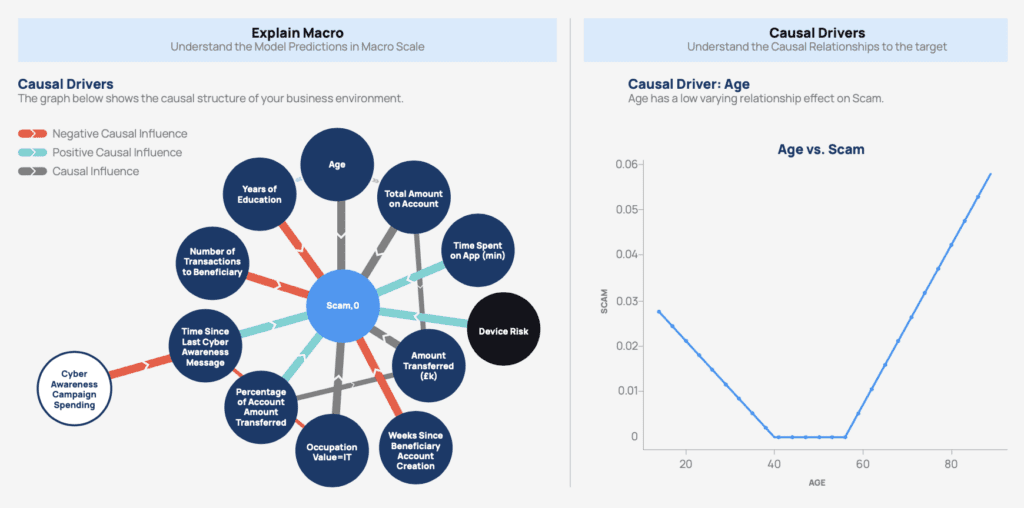
Figure 7. The system identifies the true causal drivers of fraud risk. This dashboard reveals the causal relationship between the age of the payer and their propensity to be scammed. Younger people who are new to the financial system are more susceptible than middle aged people and then susceptibility rises again over age 60.
- Enhance strategic and operational decision-making
How should you trade off the costs of payment friction from false positives versus financial loss from false negatives? What interventions or nudges can you invest in — from social media campaigns to user education tools? Causal AI goes beyond risk classifications, making intelligent recommendations of the right “threshold” at which to intervene on transactions, weighing up the implications of more risk-averse and risk-taking fraud policies and recommending the highest-ROI interventions to combat fraud.
As APP scams and other payment frauds continue to evolve at pace, the Causal Fraud Decision System provides banks with an extra layer of intelligence to out-think the fraudsters. Adopters can expect to accelerate model deployment by an order of magnitude, and reduce the total burden of fraud by roughly 70%.
The new banking AI playbook
causaLens Decision Applications have wide-ranging benefits for retail banks. They span all facets of the bank, boosting core operations, optimizing strategy, and improving customer-facing interactions. This includes: an app for stress testing that conducts explainable scenario analyses of capital and liquidity ratios under adverse economic conditions; our pricing optimizer which integrates market dynamics and changing consumer behavior to identify a competitive profit spread; and the customer retention solution, which goes beyond predicting likely customer value attrition to recommending the best actions that customer relationship managers can take.
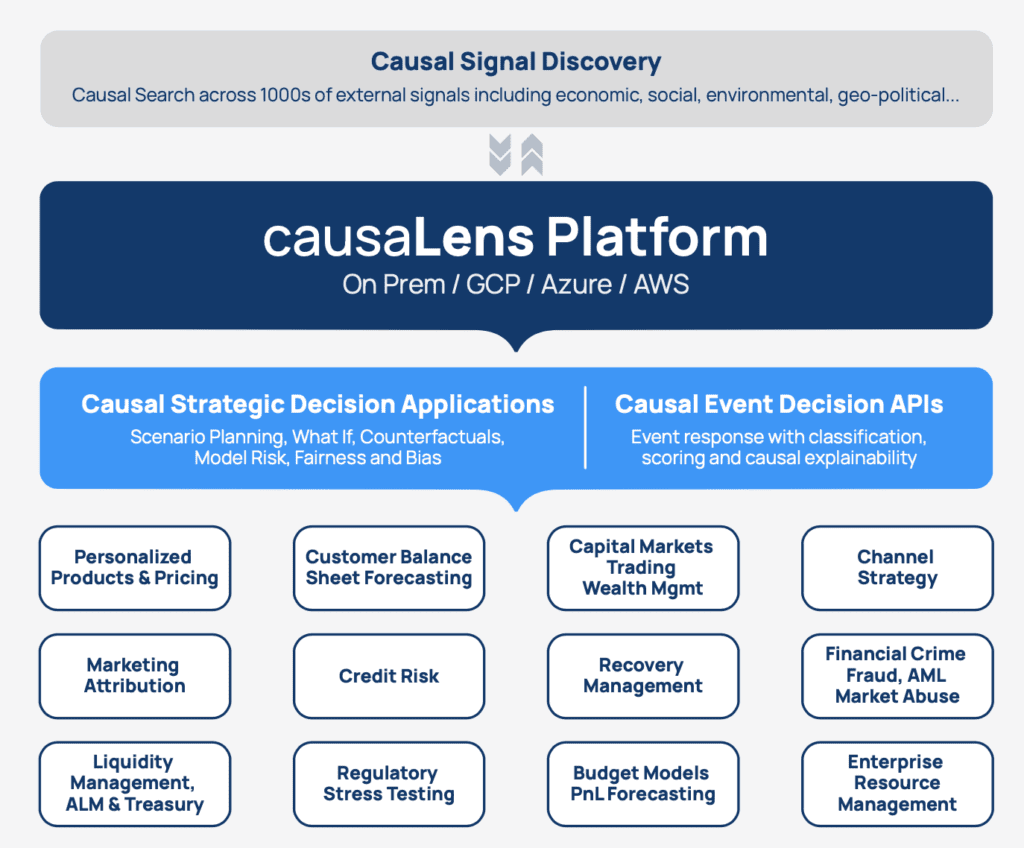
causaLens’ solutions are powered by Causal AI and built on the world’s most advanced AI Decision-Making Platform. A no-code framework enables banks to rapidly build Decision Applications for any strategic AI use case. Pre-configured components can be snapped together to rapidly build state-of-the-art solutions. Decision Applications are human-centered, based on explainable modeling, and factor in operational constraints and business context.